J. T. Mathis1, E. Osborne1, S. Starkweather2
1NOAA Arctic Research Program, Office of Oceanic and Atmospheric Research, 1315 East West Highway, Silver Spring, MD 20910, USA
2Cooperative Institute for Research in Environmental Sciences, 216 UCB, University of Colorado Boulder, Boulder, CO 8030, USA
Highlights
- Rapid environmental transitions in the Arctic highlight the need for a swift and responsive approach to scientific observing and interpretation.
- Addressing the need to produce timely and relevant scientific results, Environmental Intelligence (EI) is the production of integrated environmental knowledge from observations, modeling, and data integrations that links researchers, stakeholder and decision-makers in an iterative process.
- Application of the EI framework to an ocean acidification and Alaskan fisheries case study provides a proof of concept of the EI cycle.
Shortly after the beginning of the 21st Century, the Arctic began an environmental transition so extensive that it caught scientists, policymakers, and residents by surprise. The extent and duration of these transitions define the New Arctic, characterized by the lowest winter maximum in sea ice cover on record for 2017, the persistent and record warming of sea surface temperatures across the Arctic, and the downward trend in total ice mass of the Greenland ice sheet, just to name a few. The unprecedented rate and global reach of these changes highlight the pressing need to prepare for and adapt to the New Arctic. Historically, Arctic data have been collected through regional process studies, due to the prohibitive costs and logistical challenges of measuring at a system-wide scale. Given the urgent need for environmental information in the Arctic, scientists must now take a different approach to Arctic observing. By developing and using the Environmental Intelligence (EI) framework we aim to achieve the end-to-end integration of research across linked and iterative steps of scientific research and decision support. By integrating across Arctic system observing, data integration and modeling efforts, scientific results can be more responsive to stakeholder needs and policy-relevant. The EI approach aims to produce environmental knowledge that is timely, reliable, and suitable for decision support at the local, state, and national levels.
Environmental Intelligence Cycle
The sparseness of observations and limited seasonal coverage of in situ EI gathering has limited our ability to fully understand the dynamics of the New Arctic. Emerging challenges regarding Arctic change are globally important and include changes in Northern Hemisphere weather patterns, ecosystem and fisheries stability, sea level rise, economic development, maritime safety, and national security. To effectively study these issues, the EI framework can be applied to specific environmental issues or focused on the development of actionable environmental knowledge.
The EI Cycle (Fig. 1) starts with the identification of relevant stakeholders and decision support objectives, culminating in the determination of an environmental focus area. The next step in the cycle aims to harness existing data archives that can be leveraged to increase the spatial and temporal understanding of the environmental focus area. Identified datasets are translated into functional evidence that can then be used to identify observing gaps and to strategically enhance observing efforts. Integration of the data and observing efforts provide the best system-wide dataset available that can then be used to validate systems-level models. The modeling component of the EI cycle is critical as modeling is a vital tool to advancing system integration, capturing feedbacks within the system and projecting current trends into the future. Therefore, data integration and observing systems should focus on collecting suitable data that can enhance model outputs. The final steps of the EI cycle assess the effectiveness of new environmental knowledge for stakeholder needs and provide options for action to decision-makers.
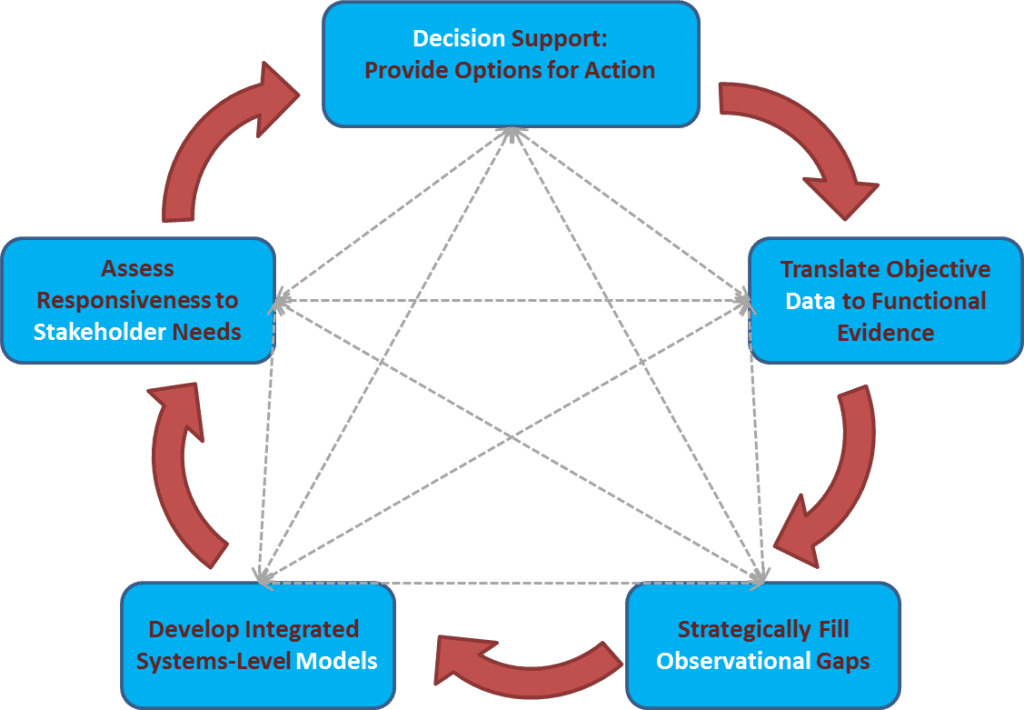
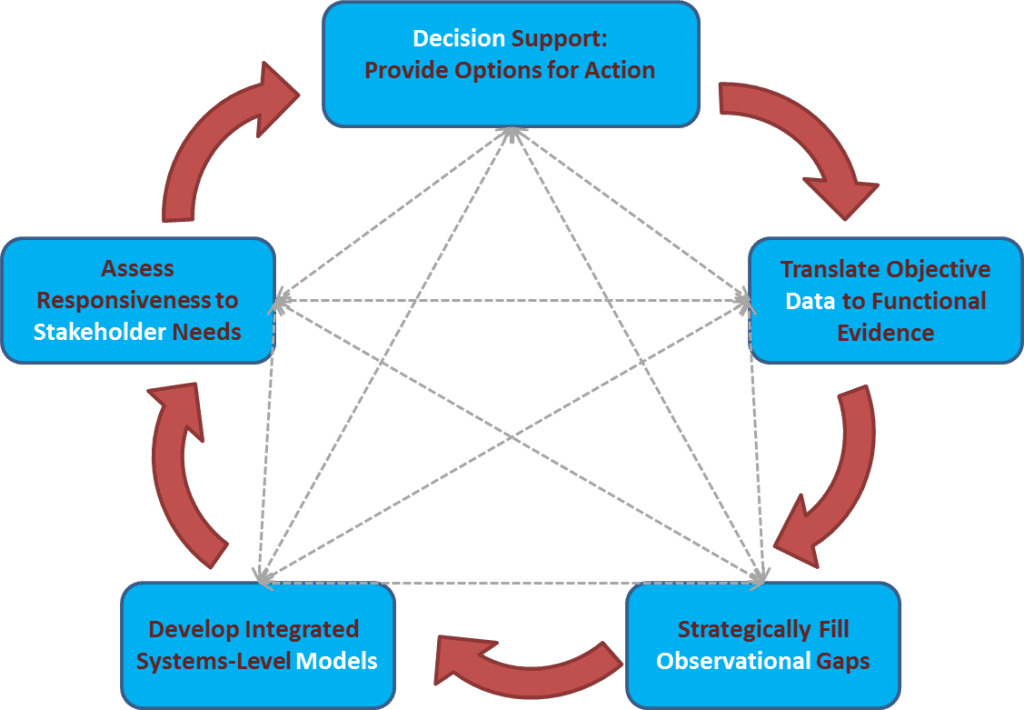
An important characteristic of the EI framework is that it is iterative, meaning the process can move quickly through its steps while still revisiting previous steps later that allow the process to be responsive to the immediate needs at hand. Specifically, the final steps of assessment and decision support often lead to new issues that need to be investigated by the research components of the EI framework. One of the most critical components of the EI construct is the seamless and continuous integration of data, observations and modeling. Disconnects between these steps can lead to inefficiencies and breakdown of the cycle as resources and outcomes become compartmentalized and not well integrated.
When possible, EI should be gathered at as many temporal and spatial scales as possible by using a nested observing framework (Fig. 2). By integrating data collection across a number of platforms—from satellites that can cover large swaths of the Arctic to community-based observers on the ground—actionable information can be gathered more efficiently. The EI Cycle prioritizes the exchange of knowledge between researchers and stakeholders, as this is critical to assessing the responsiveness of investments in data management, observing and modeling. Improvements within and across each of these areas will improve the ability to understand, communicate about, and support decisions in response to the impacts of Arctic change. These efforts, across the scales from community to global, are now of the utmost importance.
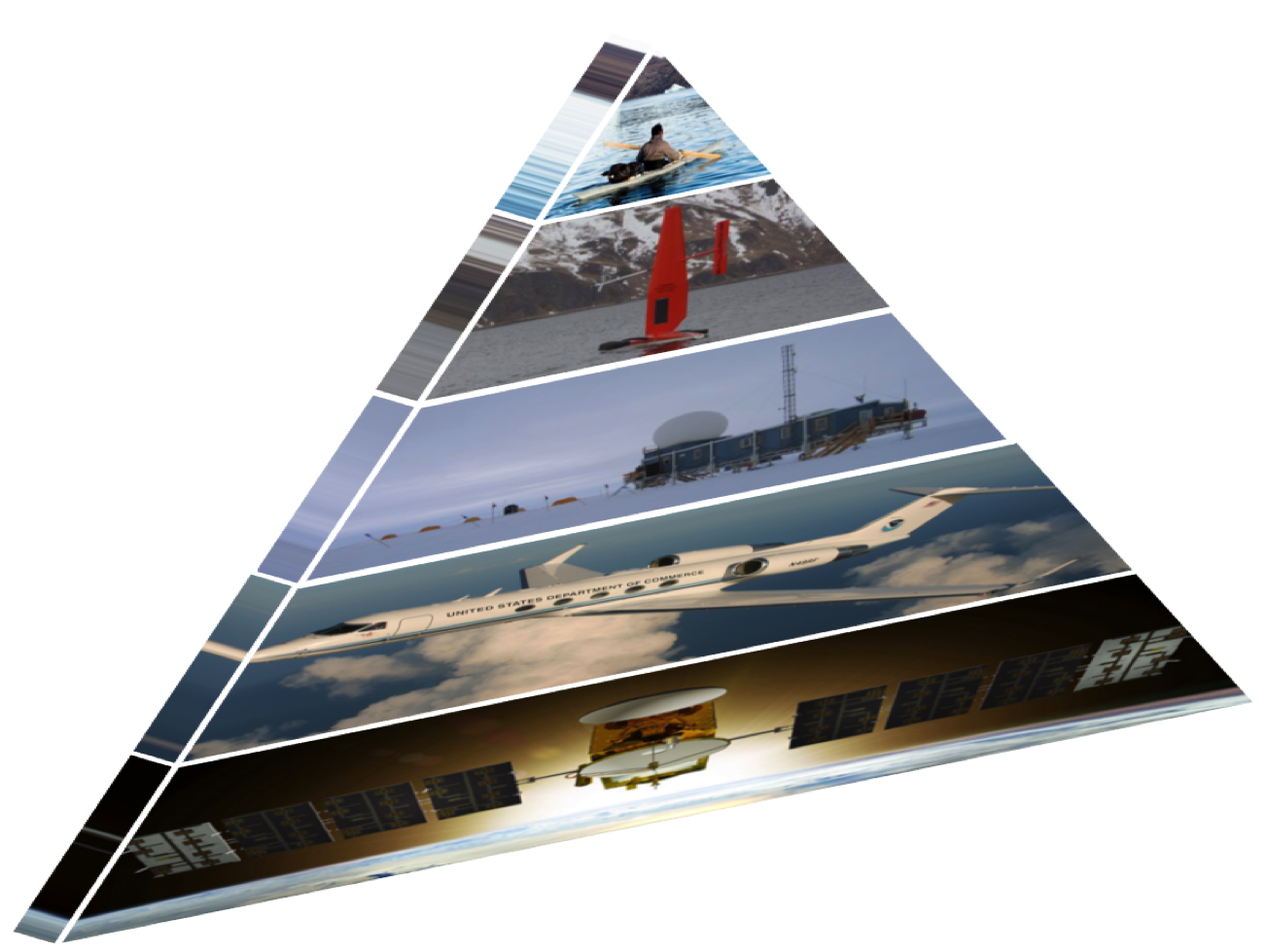
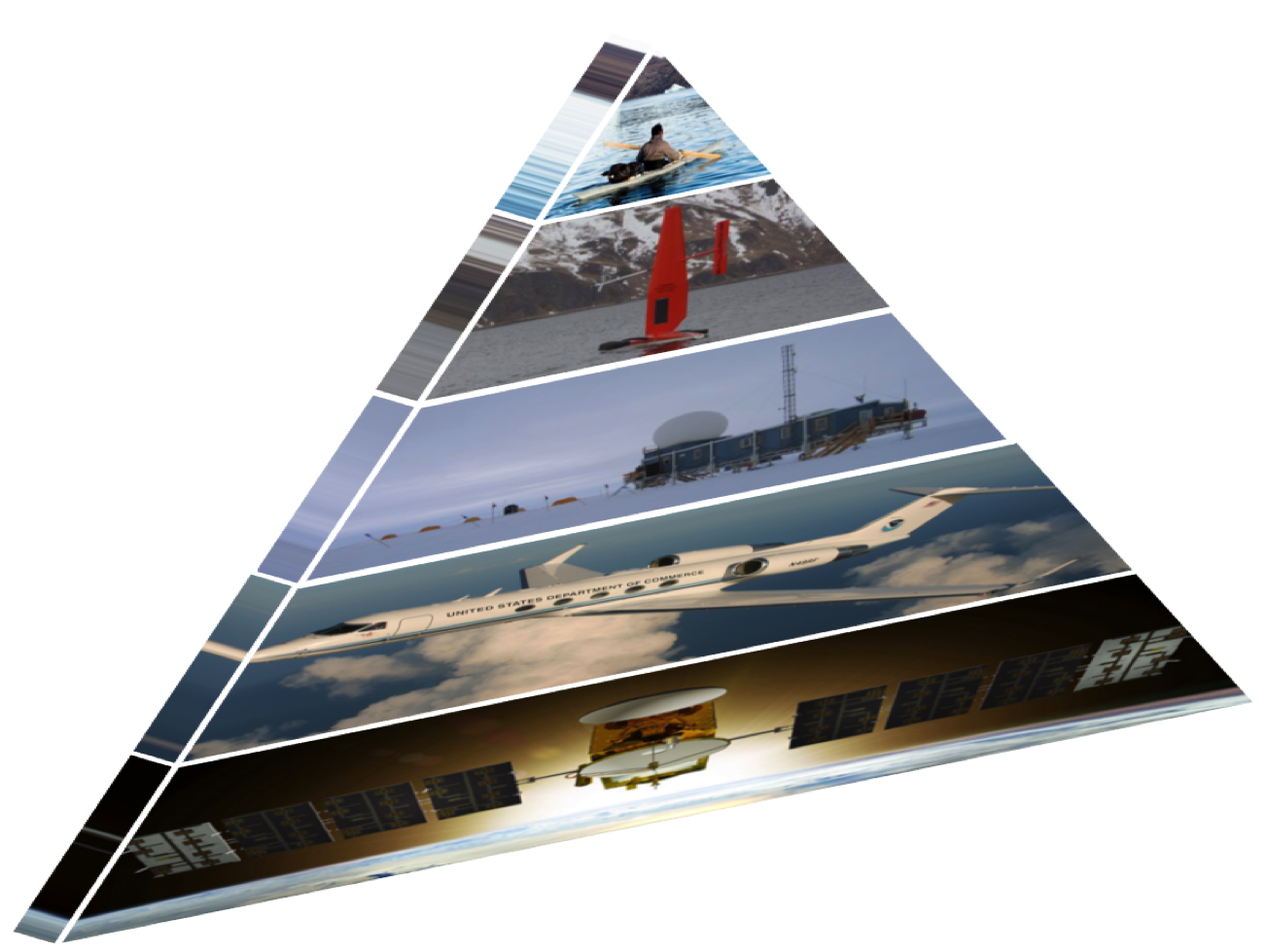
Societal Benefit Areas
The science of what is driving the changes in the Arctic is becoming clearer. However, we need to expand and improve sustained information gathering to provide actionable intelligence and forecasting needed by people of the Arctic and other stakeholders. The issues at stake include food security, cultural survival, environmental and community health as well as emerging opportunities in commerce, trade and issues of national security.
One highly effective way of doing this is to develop EI gathering around societal benefit areas (Table 1), Societal benefit areas can help frame knowledge development that is multi-disciplinary, highly relevant to stakeholder needs, and perhaps most importantly, actionable (IDA Science and Technology Policy Institute and Sustaining Arctic Observing Networks, 2017). The societal benefit areas reveal how values to society accrue the observations and value-added research efforts, like modeling and product development. As such, they can transcend national efforts, making it easier to develop functional evidence that is internationally relevant.
- Disaster Preparedness
- Environmental Quality
- Food Security
- Fundamental Understanding of Arctic Systems
- Human Health
- Infrastructure and Operations
- Marine and Coastal Ecosystems and Processes
- Natural Resources
- Resilient Communities
- Sociocultural Services
- Terrestrial and Freshwater Ecosystems and Processes
- Weather and Climate
A Case Study
To assess the effectiveness of utilizing the EI framework, it was applied to a 2014 study evaluating the effects of ocean acidification on Alaskan fisheries and economies. Ocean acidification (OA) is defined as the progressive decline in ocean pH that is caused by the accumulation of anthropogenic carbon dioxide (CO2) in seawater. The Arctic and subarctic seas are especially vulnerable to OA because a number of natural and human process act in concert to drive down pH and dissolved carbonate mineral concentrations (Mathis et al., 2015b). Alaskan Arctic organisms that strongly depend on dissolved carbonate include crabs, mussels and the low trophic-level organisms like pteropods that are an important food source for finfish such as salmon (Bednaršek et al., 2014). The vastness of areas potentially impacted by OA in the Alaskan Arctic and the associated disruptions to commercial and subsistence fisheries required a unique approach to collect environmental intelligence on this issue.
Following the EI framework, relevant stakeholders and decision support objectives were identified. In this case, a commercial shellfish hatchery in Southern Alaska needed to know if conditions were and would remain conducive for successful operation. This aligned with several key societal benefit areas (Table 1) including environmental quality, food security, marine and coastal ecosystem processes and resilient communities. This issue was also important to regional management and state and Federal policymakers due to the emergent aquaculture industry in Alaska.
The project then moved into translating objective, available data into functional evidence. In this case, there was little environmental knowledge about OA in Alaska, but there were socio-economic data that showed that Alaska seafood is a primary source of protein for 30-46% of residents of the state (Frisch et al., 2014).
With an understanding of the functional evidence, the project moved on to strategically fill observational gaps, with specific consideration as to how those observations could be used in the future step of developing systems-level models. Given the lack of actionable intelligence about OA in Alaska, an extensive observational campaign was put into place that collected millions of ocean observations at varying temporal and spatial scales. Some of these observing systems were deployed at a high intensity for a few days over a wide area (i.e., a ship-based survey), while others were deployed for several months at shore-based facilities. Observations were focused in the region adjacent to the shellfish hatchery as well as across the entire Gulf of Alaska for model development and validation activities. The data collected as a part of this observational campaign showed that OA was a chronic environmental stressor (Evans et al., 2014).
Using the new observational results, a systems-level model was developed for the region to assess the impacts of natural and anthropogenic drivers of OA that affect the Alaskan hatchery. The model outputs indicated that currently there is a 5-month window of optimal growing conditions for many juvenile shellfish species. However, model projections indicate that window is expected to close entirely by 2040 (Evans et al., 2015). The model was scaled up to a regional level for other shellfish hatcheries within the Gulf of Alaska that also needed environmental intelligence for their operations. This regional model showed similar outcomes for OA, with conditions becoming challenging for shellfish growth in hatcheries without mitigation efforts by the year 2100 (Siedlecki et al., 2017).
Completing the EI Cycle, we returned to the initial step of our EI case study to determine responsiveness to stakeholder needs. A fully developed risk assessment was established for Alaskan fisheries using the environmental knowledge gathered as a part of this study. These new insights showed that Alaskan commercial and subsistence fisheries are located in seas projected to experience rapid transitions in temperature, pH, and other chemical parameters caused by global change, especially OA. Many of the marine organisms that are most intensely affected by OA, such as mollusks, contribute substantially to the state’s highly productive commercial fisheries and traditional subsistence way of life (Mathis et al., 2015a).
The outcome of this first trip through the EI cycle did address the needs identified at the outset of the EI Cycle and supported a number of societal benefit areas. However, the results also identified more decision support areas, which is exactly how the EI Cycle is supposed to work. In this case, from start to finish, the first iteration of the EI Cycle took approximately 18 months, but the project continues today as more environmental knowledge is generated. The faster a project can move through each round of the EI Cycle, the more responsive it can be to both identifying and addressing stakeholder needs and societal benefits.
Final Thoughts
Global connections to Arctic change are becoming more pronounced, including sea level rise and the propagation of extreme weather events into lower latitudes. It is clear that the New Arctic brings challenges as well as opportunities. In recent years, the Arctic has undergone unprecedented transitions and trends show a clear, stronger and more pronounced signal of persistent warming than at any point in our observational record to at least the late 1800s. The rate and magnitude of these changes challenge the Arctic community to expand and improve sustained information gathering to more effectively provide actionable intelligence and forecasting needed by residents and stakeholders. As shown in the OA case study, the use of the EI Cycle is a highly effective way to respond to the rapid transitions that are underway. Other issues at stake that could benefit from the EI framework include food security, cultural survival, and community health, along with emerging opportunities in commerce and trade.
References
Alessa, L., A. Kliskey, J. Gamble, M. Fidel, G. Beaujean, and J. Gosz, 2015: The role of Indigenous science and local knowledge in integrated observing systems: moving toward adaptive capacity indices and early warning systems. Sustain Sci., 11, 91-102, doi: 10.1007/s11625-015-0295-7.
Bednaršek, N., G. A. Tarling, D. C. E. Bakker, S. Fielding, and R. A Feely, 2014: Dissolution dominating calcification process in polar pteropods close to the point of aragonite undersaturation. PLoS One, Vol. 9, Issue 10.
Evans, W., J. T. Mathis, and J. N. Cross, 2014: Calcium carbonate corrosivity in an Alaskan Inland Sea. Biogeosciences, 11, 365–379, doi: 10.5194/bg-11-365-2014.
Evans, W., J. T. Mathis, J. Ramsey, and J. Hetrick, 2015: On the frontline: Tracking CaCO3 corrosivity in an Alaskan shellfish hatchery. PLoS One, 10(7), e0130384, doi: 10.1371/journal.pone.0130384.
Frisch, L. C., J. T. Mathis, N. P. Kettle, and S. Trainor, 2014: Gauging perceptions of ocean acidification in Alaska. Marine Policy, 53, 101-110, doi: 10.1016/j.marpol.2014.11.022.
IDA Science and Technology Policy Institute and Sustaining Arctic Observing Networks. 2017: International Arctic Observations Assessment Framework. IDA Science and Technology Policy Institute, Washington, DC, U.S.A., and Sustaining Arctic Observing Networks, Oslo, Norway, 73 pp.
Mathis, J. T., S. R. Cooley, N. Lucey, S. Colt, J. Ekstrom, T. Hurst, C. Hauri, W. Evans, J. N. Cross, and R. A. Feely, 2015a: Ocean acidification risk assessment for Alaska’s fishery sector. Progress in Oceanography, 136, 71-91, doi: 10.1016/j.pocean.2014.07.001.
Mathis, J. T., J. N. Cross, W. Evans, and S. Doney, 2015b: Ocean acidification in the surface waters of the Pacific-Arctic boundary regions. Oceanography, 28(2), 122-135, doi: 10.5670/oceanog.2015.36.
Siedlecki, S. A., D. J. Pilcher, A. J. Hermann, K. Coyle, and J. Mathis, 2017: The importance of freshwater to spatial variability of aragonite saturation state in the Gulf of Alaska. Journal of Geophysical Research: Oceans, 122, https://doi.org/10.1002/2017JC012791.
January 5, 2018